Classifying emotions through multimodal signal recordings with Empatica E4
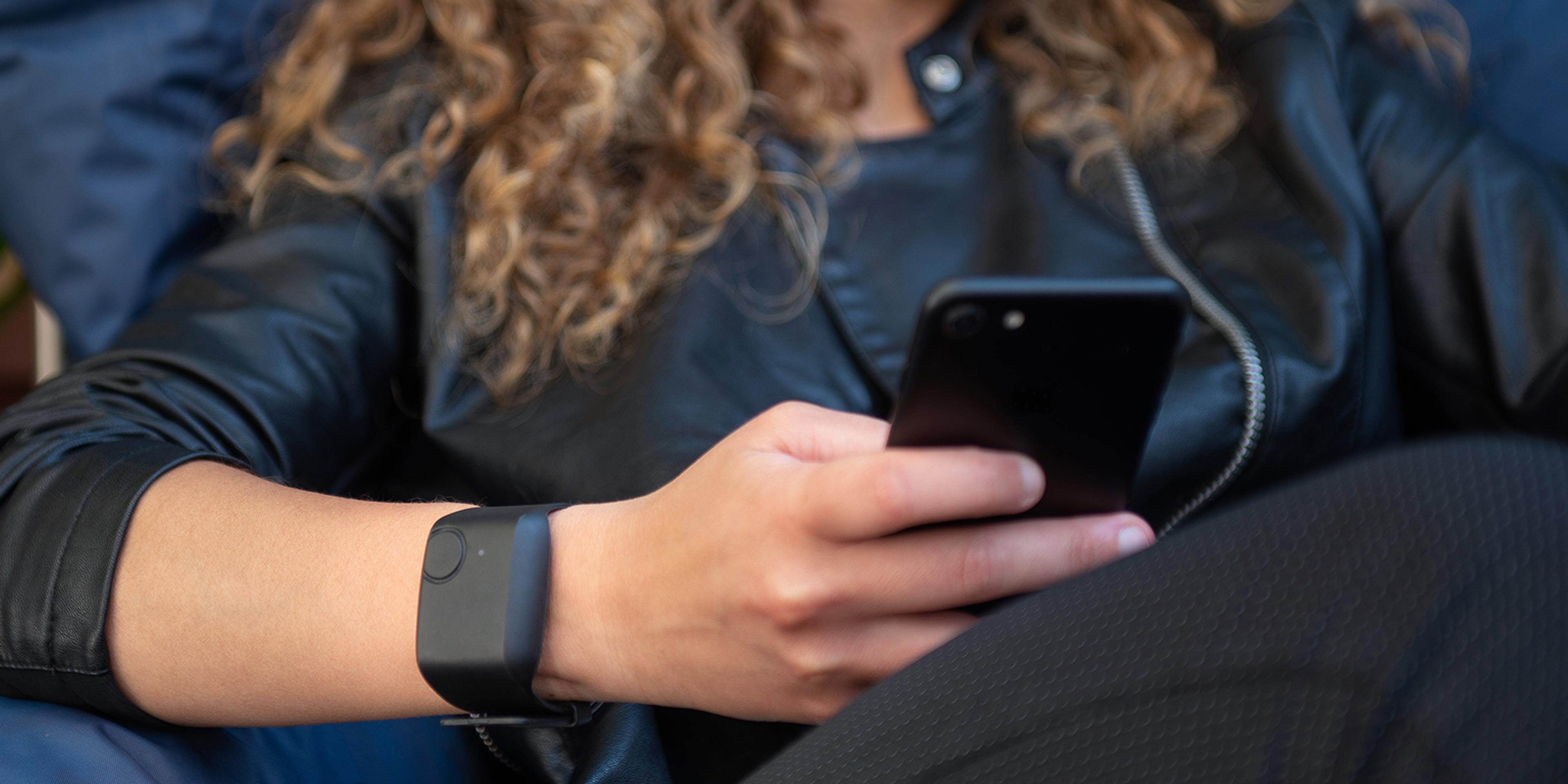
This article has been guest-authored by Gloria Cosoli, PhD, Post-Doctoral Research Fellow at the Department of Industrial Engineering and Mathematical Sciences of Università Politecnica Delle Marche, Ancona, Italy. Her research targets non-invasive measurement systems and methods for different application fields, such as Biomedics, Construction, and Mechanics, along with numerical simulations and signal processing techniques. In the research field of wearable devices, she is particularly focused on metrological aspects, aimed at quantifying the device's measurement accuracy and precision in different application fields, such as healthcare, domestic well-being, and Industry 4.0.
The presence of stimuli undoubtedly reflects on physiological signals changes. Nowadays, the wide application of wearable devices has made the continuous monitoring of vital signs by user-friendly wrist-worn devices like Empatica E4 (medical graded device according to the 93/42/EEC Directive) common.
This blog post draws on the results of the study titled “Measurement of multimodal physiological signals for stimulation detection by wearable devices”, which was published in August 2021 in the Measurement journal.
Emotion detection and recognition feed an active research field, involving many different applications, such as safe driving, healthcare, and social security [1]. Plutchick [2] defined a taxonomy based on 8 emotions, namely joy, trust, fear, surprise, sadness, disgust, anger, and anticipation. Multi-dimensional space models were then developed, considering valence (pleasant vs unpleasant), arousal (high vs low), and, later on, dominance (submissive vs dominant).
What are emotions, and how can we objectively assess them?
- An emotion can be thought of as a subject’s reaction to a stimulus. This provokes a change in the subject’s physiological state and, consequently, will be detectable through variations in vital signs. The sympathetic nervous system (SNS) controls these fluctuations, which cannot be regulated voluntarily, contrarily to facial/behavioral appearance. Such objective modifications can be revealed through a plethora of sensing technologies: electrocardiogram (ECG), electroencephalogram (EEG), electromyogram (EMG), photoplethysmogram (PPG), electrodermal activity signal (EDA), and skin temperature (SKT). Being a multisensor wearable device, Empatica E4 allows multimodal physiological signals acquisition and analysis, which provides many advantages:
- A broader fingerprint of the subject’s physiological state [3], depicting different aspects that could not be described through a single sensor measurement;
- Inclusion of physiological inter-subject variability commonly affecting vital signs and emotions;
- Improvement in the motion artefacts identification and, hence, enhancement of data quality.
Emotions obviously affect a subject’s physiological state and well-being. In this context, it is worthy to underline that nowadays the healthcare paradigm is shifting towards remote health monitoring, telemedicine, and home care. Wearable devices undoubtedly play a pivotal role, also for their IoT-enabled capacities of data sharing in real-time. On the other hand, the rapid growth of the amount of data collected through wearables has pushed the application of Artificial Intelligence (AI) to the analysis of the so-called “big data”, providing potentially useful information to support decision-making processes, especially in the healthcare context. However, depending on the target application, it is fundamental to properly consider the metrological performance of the whole measurement chain: both hardware sensors and software contribute with their own uncertainties, thus determining the output accuracy and the reliability of related decisions [4].
Method
The study, performed at Università Politecnica Delle Marche (Italy) as a joint collaboration between the Department of Industrial Engineering and Mathematical Sciences and the Department of Information Engineering, involved 7 healthy volunteers. Signals were recorded at rest, with the participants wearing the Empatica E4 on the dominant wrist while reproducing 1-minute audio recordings as stimuli (selected from the International Affective Digitized Sound system, IADS-2, database [5]). Three different events (neutral, pleasant, and unpleasant) were chosen and proposed twice to each subject, making a total of 42 recordings. The event-marker button of Empatica E4 was used to timely label the beginning/end of each stimulus.
The following signals were considered for the analysis:
- Blood Volume Pulse (BVP); obtained through the PPG sensor (employing 4 LEDs and 2 photodiodes, with a sampling frequency of 64 Hz) and through which Heart Rate Variability (HRV, i.e. the physiological variability in the duration of the heartbeat) was derived. HRV analysis was performed through the Kubios software tool [6];
- Electrodermal activity (sampled at 4 Hz through Ag/AgCl electrodes); reflecting the eccrine sweat gland activity and, hence, quantifying the “emotional sweating”. Particular attention should be paid to interfering inputs, such as electronic noise and variations in skin-electrode contact. EDA processing was performed with the Biosignal-Specific Processing (Bio-SP) Tool [7] from Matlab;
- Skin temperature (measured through a thermopile with a sampling frequency of 4 Hz and an accuracy of ±0.20 °C), which can vary with changes in blood flow determined by SNS activity on smooth muscle tone.
All these quantities have been previously employed in literature for emotion recognition and stress detection. Given that arousal is said to affect EDA signal more than valence, the authors opted for a binary classification between stimuli presence/absence, with an accuracy that should be superior with respect to valence differentiation. Then, the previously trained algorithms were validated on a different publicly available dataset, called the Wearable Stress and Affect Detection (WESAD) multimodal dataset [8], considering daily external stimuli, hence widening the application of the proposed method.
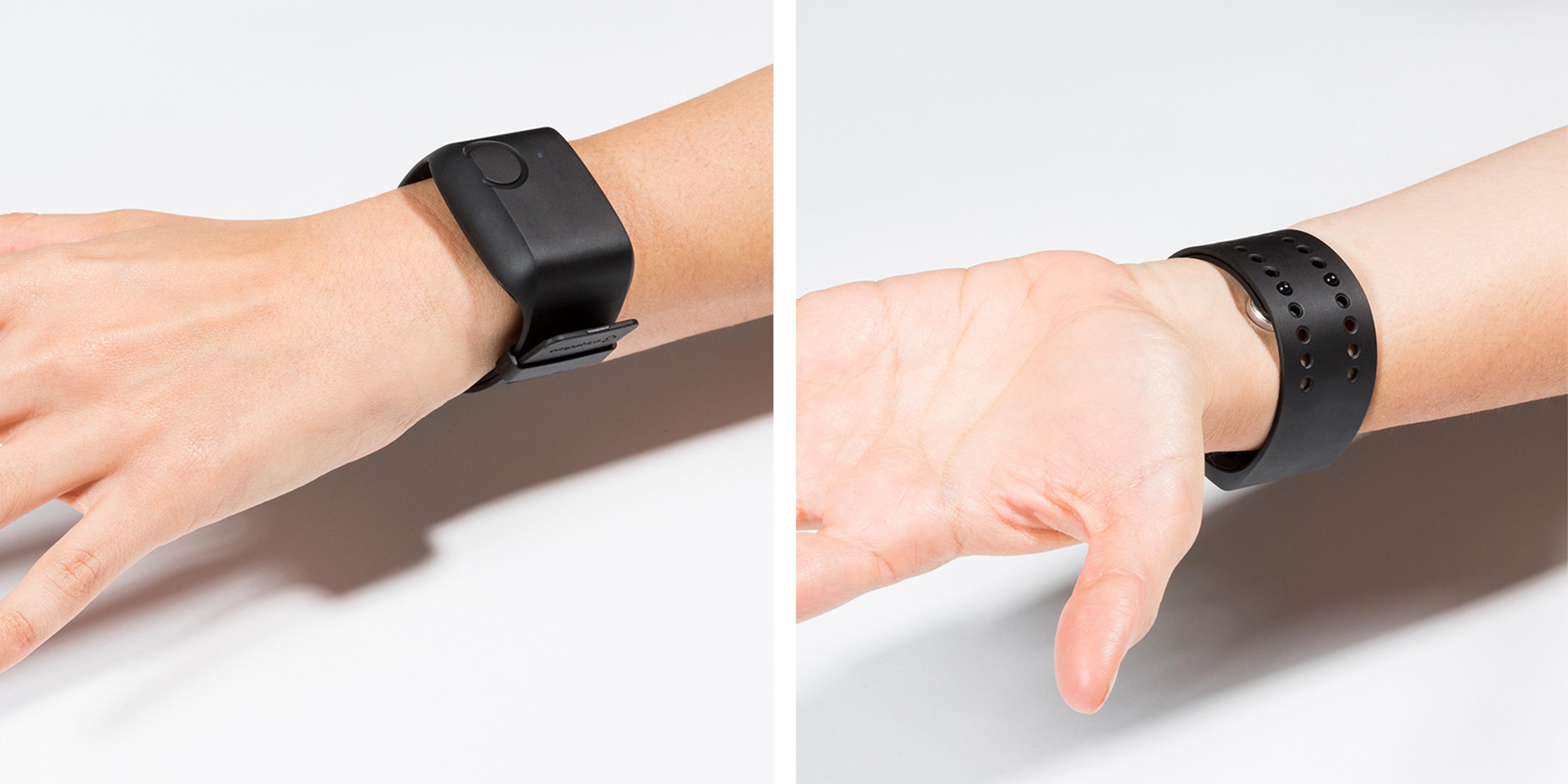
Data processing and Machine Learning classification
An unquestionable advantage of Empatica E4 is the possibility to access the acquired raw data; this allows for several types of data processing that would not be possible on synthetic data (which, on the contrary, are provided by most of the current commercial wearable devices).
The data processing pipeline consisted of 4 main steps:
- Labeling: data were categorized as presence/absence of stimuli;
- Filtering: data were properly filtered according to literature recommendations to improve the Signal-to-Noise Ratio (SNR);
- Feature extraction: significant metrics in both time and frequency domains were extracted;
- Feature selection: the correlation-based algorithm[9] was employed to avoid redundant and irrelevant features and improve classification performance; 22 features out of 33 were maintained.

Different machine learning (ML) algorithms were tested for binary classification between stimuli presence/absence: Random Forest, Decision Tree, Naïve Bayes, K-nearest neighbor, Bagging, Boosting, Support Vector Machine (SVM), and Linear Regression (LR). The 10-fold cross-validation method was used, considering accuracy as the average over 10 iterations. Classification performance was evaluated through standard metrics, namely accuracy, sensitivity, precision, and F-measure[10].
Results
LR algorithm achieved an accuracy of 75%, followed by SVM with an accuracy of 72.62%; this confirms the possibility of stimulus elicitation recognition through multimodal signals analysis acquired by a wearable device. In the WESAD validation, the discrimination performance was equal to 71.43% and 64.29% for LR and SVM, respectively, proving that the previously trained algorithms can be applied also for the detection of different stimuli.
Conclusions
Stimulation causes reactions to a subject’s physiological state. Hence, identifying the presence/absence of stimuli can help to take timely actions aimed at optimizing the subject’s well-being status, whenever possible.
Wearable devices can be used to continuously record physiological signals depicting the subject’s state, and it is better if multiple signals can be simultaneously acquired, like in the case of Empatica E4. Photoplethysmographic signal-related features appear to be the most influential in the discrimination between presence/absence of stimuli; however, a multimodal system allows a wider context description, minimizing the effect of noise and artefacts.
On the other hand, Machine Learning tools appear successful in the classification, thus supporting decision-making processes, potentially useful in different contexts, such as healthcare and Industry 4.0, where the big data collection results pivotal to properly consider physiological variability.
Credit
The scientific article “Measurement of multimodal physiological signals for stimulation detection by wearable devices” was authored by Gloria Cosoli, Angelica Poli, Lorenzo Scalise, and Susanna Spinsante.

References
Paper: Cosoli, G., Poli, A., Scalise, L., & Spinsante, S. (2021). Measurement of multimodal physiological signals for stimulation detection by wearable devices. Measurement, 184, 109966. https://doi.org/10.1016/j.measurement.2021.109966
[1] L. Shu, et al., A review of emotion recognition using physiological signals, Sensors (Switzerland) 18 (7) (2018).
[2] R. Plutchik, The Nature of Emotions: Human emotions have deep evolutionary roots, a fact that may explain their complexity and provide tools for clinical practice, in Am. Sci. 89, Sigma Xi, The Scientific Research Honor Society, 2001, pp. 344–350.
[3] Y. Dai, X. Wang, P. Zhang, W. Zhang, Wearable biosensor network enabled multimodal daily-life emotion recognition employing reputation-driven imbalanced fuzzy classification, Measurement 109 (2017) 408–424.
[4] Casaccia, S., Revel, G. M., Cosoli, G., & Scalise, L. (2021). Assessment of domestic well-being: from perception to measurement. IEEE Instrumentation & Measurement Magazine, 24(6), 58-67.
[5] M.M. Bradley, P.J. Lang, “The International Affective Digitized Sounds (2nd Edition; IADS-2), Affective Ratings of Sounds and Instruction Manual.” NIMH Center for the Study of Emotion and Attention (2007).
[6] “Biosignal Analysis and Medical Imaging Group - Kubios HRV.” [Online]. Available: http://kubios.uef.fi/.
[7] S. Ostadabbas, “Biosignal-Specific Processing (Bio-SP) Tool.” (https://www.mathworks.com/matlabcentral/fileexchange/64013-biosignal-specific-processingbio-sp-tool), MATLAB Central File Exchange. Retrieved November 27 (2020).
[8] P. Schmidt, A. Reiss, R. Duerichen, C. Marberger, K. Van Laerhoven, “Introducing WESAD, a Multimodal Dataset for Wearable Stress and Affect Detection,” in Proceedings of the 20th ACM International Conference on Multimodal Interaction (2018) 400–408.
[9] N. Gopika, A. M. Kowshalaya M.E., “Correlation Based Feature Selection Algorithm for Machine Learning,” in 2018 3rd International Conference on Communication and Electronics Systems (ICCES) (2018) 692–695.
[10] D.J. Cook, Activity learning : discovering, recognizing, and predicting human behavior from sensor data, John Wiley & Sons, 2015.