E4 Bytes: Developing a model to predict migraine attacks using biosignals
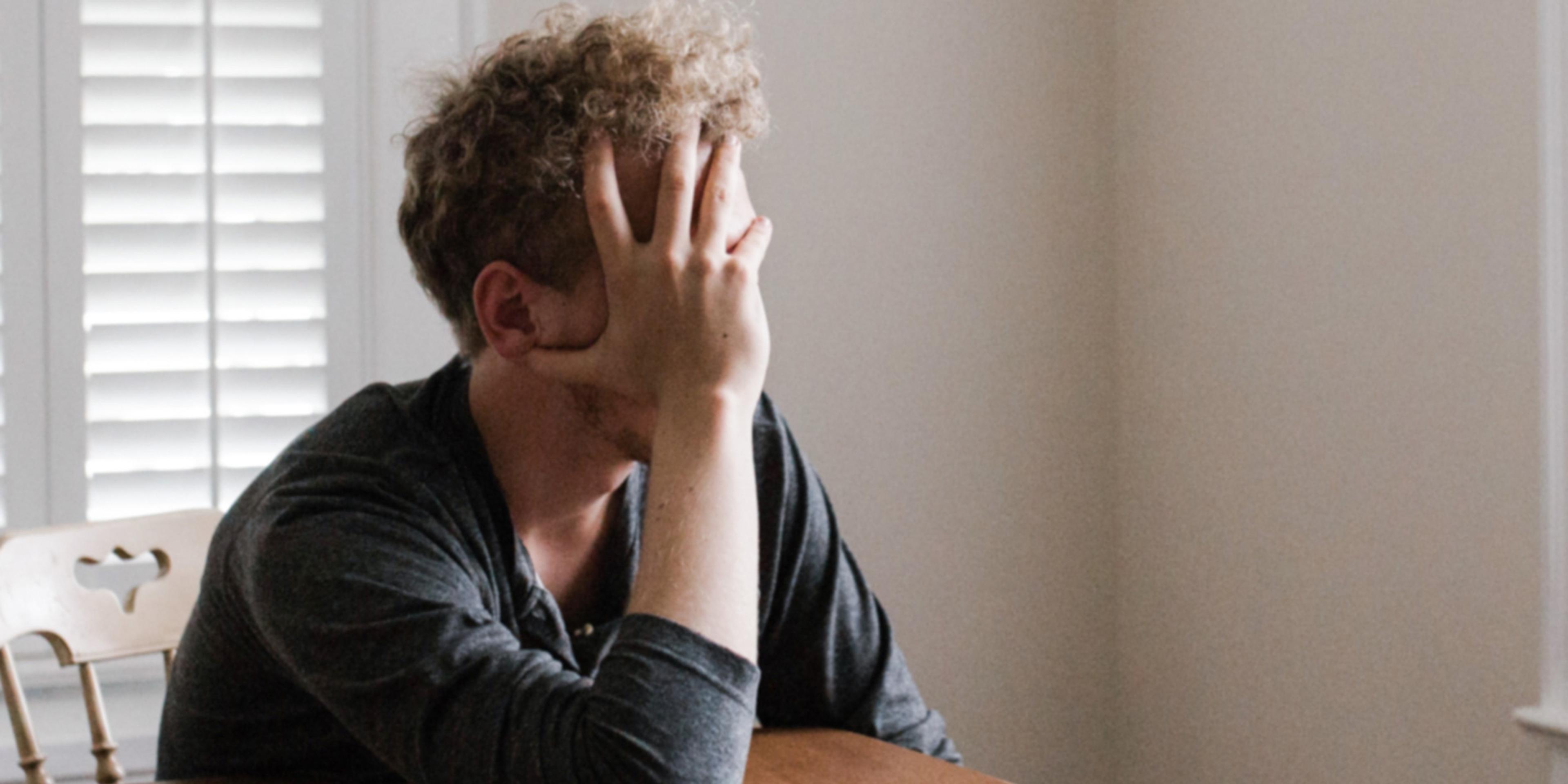
Researchers at the University of Oulu describe how they used the E4 to develop a model that predicts migraine attacks using biosignals.
The migraine is a chronic, incapacitating neurovascular disorder, characterized by attacks of severe headache and autonomic nervous system dysfunction, concerning 15% of people in developed countries. It is one of the most understated and incapacitating diseases in the world and costing yearly 111 Billion Euros in Europe only. However, the driving force to start this study was Heli’s son who started to have migraine attacks since he was 2-year-old.
The focus was on early detection of the attacks by using human measured biosignals. Empatica E4 was selected to be used in the study as it is the most capable device in the market, containing more sensors than any other wrist-worn device, and it has been found reliable in the previous studies as well. By using it, the aim was to develop a model that assists individuals to take their medication on time and hence help to avoid the pain in migraine.
Our first article [1] concentrated on the data gathering experiences of 11 volunteers. They had reported having migraine attacks at least twice in a month, and asked to wear the E4 24/7 for approximately one month. In our second article [2], the gathered data were used to train machine learning models for the early detection of migraine attacks. It was decided to concentrate on analyzing sleep time data as it contains much less disturbances caused by movement than day time data. The idea of this approach was to tell the user when he/she wakes up in the morning if he/she is going to have a migraine attack that day and take medication if needed.
Altogether, 110 features were extracted from each night’s biosignals, and used to train machine learning models. The experiments showed that early symptoms for migraine are highly personal. In fact, the balanced recognition accuracies using user-independent models were below 50%. It was shown that using personal recognition models the accuracy for detecting attacks one night prior is over 84%. While the number of study subjects was small and there is a lot of variation in the detection rates between the study subjects, this shows the potential of the introduced approach and Empatica E4.
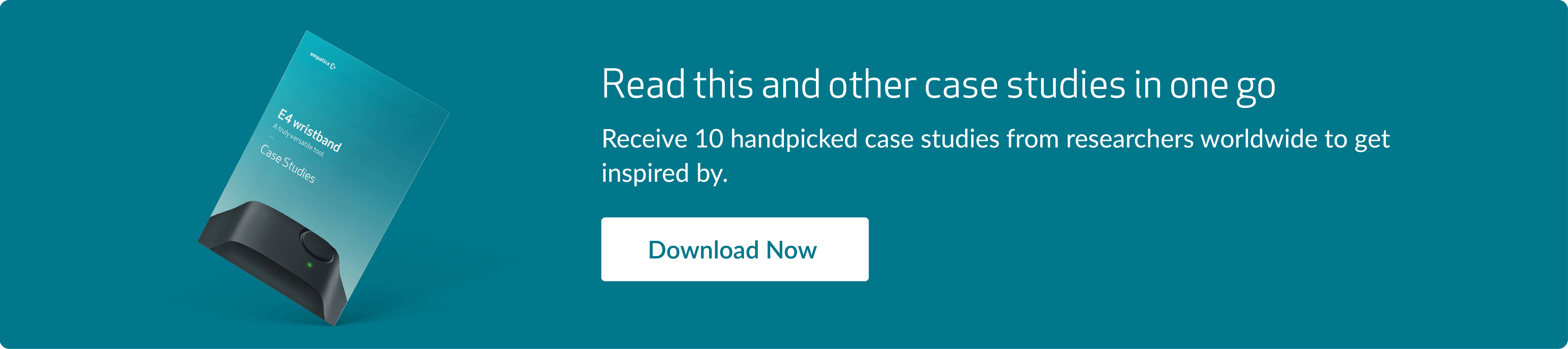
References:
[1] Koskimäki, H., Mönttinen, H., Siirtola, P., Huttunen, H. L., Halonen, R., & Röning, J. (2017). Early detection of migraine attacks based on wearable sensors: experiences of data collection using Empatica E4. In Proceedings of the 2017 ACM International Joint Conference on Pervasive and Ubiquitous Computing and Proceedings of the 2017 ACM International Symposium on Wearable Computers (pp. 506-511).
[2] Siirtola, P., Koskimäki, H., Mönttinen, H., & Röning, J. (2018). Using sleep time data from wearable sensors for early detection of migraine attacks. Sensors, 18(5), 1374.